TV-UDA: ThermalVisible Unsupervised Domain Adaptation
2 Enova Robotics, 4023, Sousse, Tunisie;

The TE-GAN framework. The proposed architecture of adaptive object detection via adversarial learning. The adaptation process is performed by means of multi-level feature alignments guided by an uncertainty measure. It also incorporated consistency and contextual regularization modules.
Highlights
- The proposed adaptation architecture has the advantage of performing detection in the target domain at no additional annotation cost.
- The proposed adaptation scheme for detection has the advantage of leveraging information from the labeled source domain to deal with the gap in the target domain at no additional annotation cost. %without supervision %in the target domain.
- To cover different aspects of the domain shift, multi-level feature alignments guided by an uncertainty measure are performed.
- The adaptation process also incorporates consistency and contextual regularization modules.
- To cover different aspects of the domain shift, we propose in this paper a more architecture compared to the state-of-the-art methods, by performing multi-level feature alignments guided by an uncertainty measure. The adaptation process also incorporates consistency and contextual regularization modules.
- By means of tests, the adaptability power of the proposed detector is proven in different domain shift scenarios.
Abstract
Unsupervised domain adaptation is a promising solution to adapt models to new domains or environments at no additional annotation cost. Such adaptation strategy has been investigated for detection to cope with the domain shift along the detection pipeline. To improve the transferability of object detectors between source and target domains, we propose in this paper to perform multi-level feature alignments via adversarial learning. The alignment process is guided by an uncertainty measure to adapt weights of well-aligned and misaligned target samples, accordingly. Furthermore, the proposed approach includes a consistency regularization to enforce the coherency between image and instance level domain classifiers. We also emphasize the need for leveraging contextual information that captures the scene structure for complementary aspects and to further improve the adversarial training process. These adaptation components are integrated into Faster R-CNN architecture at different levels. The resulting proposed adaptive detector has the advantage of covering different aspects of the domain shift in order to improve the overall performance. Extensive experiments of different domain shift scenarios demonstrate the effectiveness of our proposed adaptation scheme by obtaining better results compared to the baseline detector and to the current state-of-the-art adaptive detectors.
Results
1. Qualitative Results

2. GradCam gradient visualization.
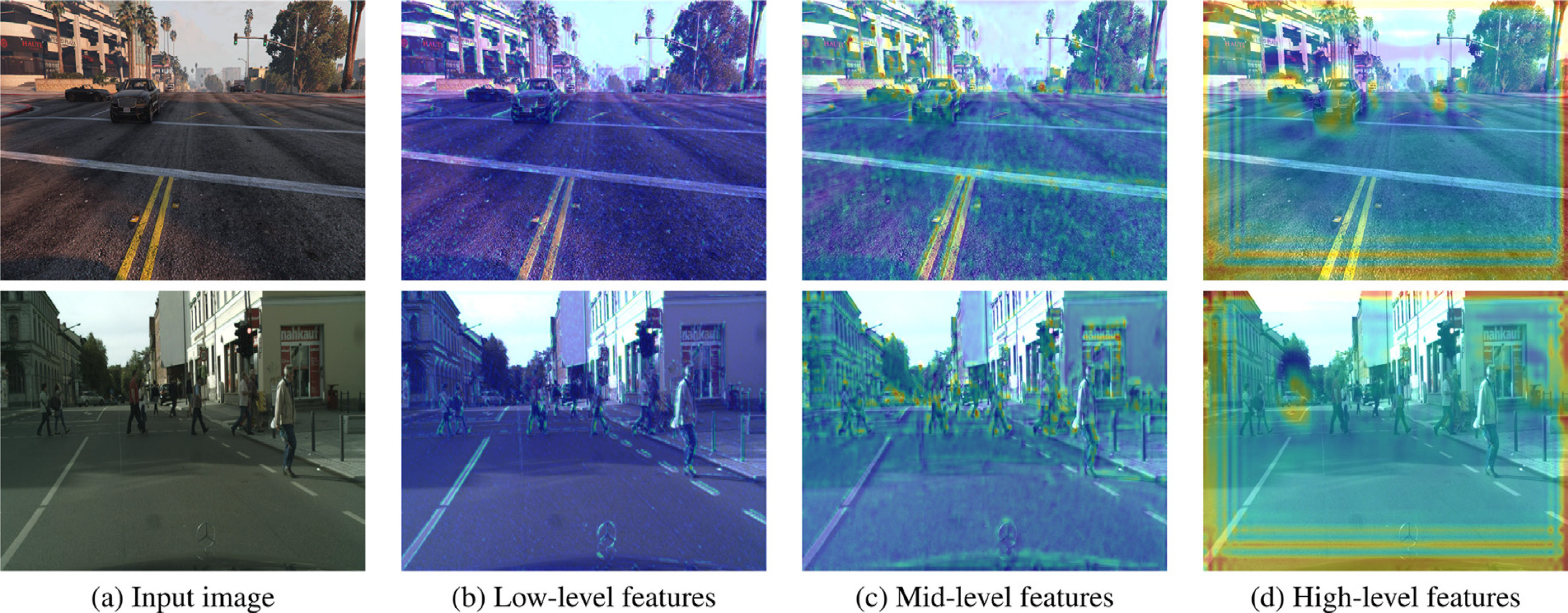
3. Feature visualization.
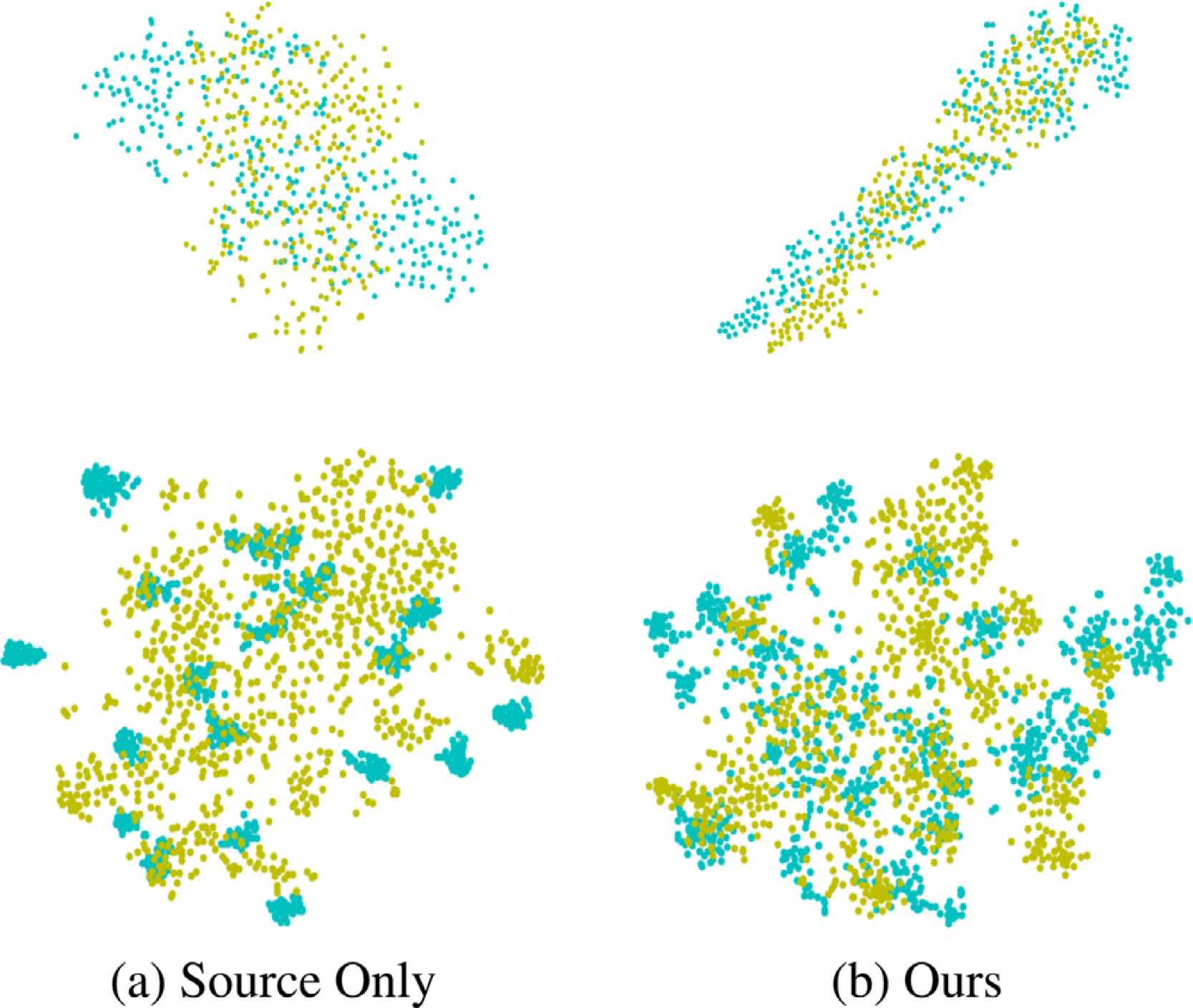
Citation
@article{marnissi2023improved, title={Improved domain adaptive object detector via adversarial feature learning}, author={Marnissi, Mohamed Amine and Fradi, Hajer and Sahbani, Anis and Amara, Najoua Essoukri Ben}, journal={Computer Vision and Image Understanding}, pages={103660}, year={2023}, publisher={Elsevier} }
Contact
If you have any question, please contact Mohamed Amine Marnissi at mohamed.amine.marnissi@gmail.com.